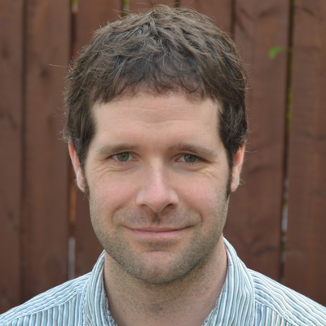
Dr. Philippe Després uses high-performance computing to generate and analyze medical images — most of them from cancer patients — and develops digital tools for clinicians and other health professionals.
“Representing the anatomy in 3D requires the acquisition of images that encompass 360 degrees of the patient,” says Dr. Després, a Professor in the Department of Physics, Engineering Physics and Optics at Université Laval and a Medical Physicist at CHU de Québec-Université Laval, one of Canada’s largest academic hospitals. “The 3D image is obtained by a mathematical process called tomographic reconstruction, which can be quite demanding from a computational perspective. We accelerated this kind of algorithm to get better images in time frames compatible with clinical activities and that means we have to provide them very rapidly.”
Another key aspect of medical imaging is related to the radiation dose.
“There are hundreds of CT scans made in Canada each day” he says. “It is important to use radiation wisely and to aim for doses as low as possible in medical imaging. The iterative reconstruction algorithms we develop contribute to reduce this dose while preserving the quality of images.”
In his work, Dr. Després uses graphics processing units (GPU) to accelerate computation tasks. These devices, originally designed for video games, help him speed up algorithms by factors that can reach 100 or 1,000 — a game-changer in clinical and other sectors.
Dr. Després succeeded in accelerating dose calculations in high magnetic fields, and he collaborated with an industrial partner in the development of a new device in radiation oncology capable of treating cancer while imaging the anatomy with MRI.
“Dose calculations in magnetic fields took a long time before the advent of GPU” Dr. Després says, adding that his group’s work contributed to making the new device a clinical reality, with calculations taking minutes instead of days. “That’s as different as night and day in terms of clinical viability,” he says. “Although you might have a very nice algorithm, if it takes an hour to run, it’s not compatible with clinical workflows. If you can accelerate it by a factor of 1,000 with GPUs, then it becomes useful.”
Dr. Després is also involved in the development of digital infrastructures and is passionate about research data management (RDM).
“As researchers, we are handling a lot of data, but we are often ill-equipped to manage it properly,” he says. “My interest in research data management has grown enormously over the past two years, and I began to implement RDM good practices in my lab, including the FAIR principles, stating that data should be findable, accessible, interoperable and reusable. Medical physicists are probably the geekiest people in the hospital, so it’s natural for us to make sure the data are managed and used properly”.
My biggest challenge is “to convince people that research data management is important. Funding agencies are beginning to realize this; reviewers of grants are becoming increasingly aware. We have to make it clear that RDM good practices are an essential component of excellence in research. Generating data costs a lot and you don’t want to do it twice. If a public agency is funding my project, then associated data isn’t mine, it belongs to the community. This is a significant challenge as it entails a culture change.”
I had to come to terms with “the fact that AI became a buzzword. Everybody wants to do AI, but few have the high-quality data required to do it properly, especially in health.”
My message to others is “take the time to consider and learn about RDM. Take the time to familiarize yourself with best practices, making sure data is findable, accessible, interoperable and reusable. You’ll see that your research will benefit from it.”